Researchers developed a novel algorithm to solve data assimilation problems using quantum computers, significantly reducing computational cost
Data assimilation is an important mathematical discipline in earth sciences, particularly in numerical weather prediction (NWP). However, conventional data assimilation methods require significant computational resources. To address this, researchers developed a novel method to solve data assimilation on quantum computers, significantly reducing the computation time. The findings of the study have the potential to advance NWP systems and will inspire practical applications of quantum computers for advancing data assimilation.
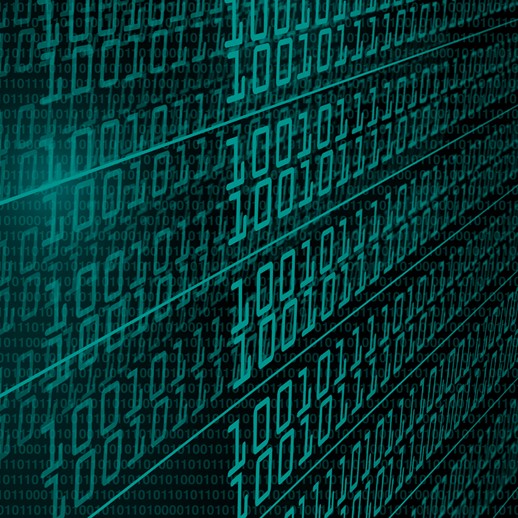
Image title: Quantum computer-aided calculation
Image caption: The novel quantum data assimilation method can significantly reduce the computation time required for numerical weather prediction, enabling deeper understanding and improved predictions
Image credit: Brett Jordan from Openverse
https://openverse.org/image/563410ca-1385-475c-a7f6-fd521f910623
Image license: CC BY 2.0
Usage restrictions: Credit must be given to the creator.
Data assimilation is a mathematical discipline that integrates observed data and numerical models to improve the interpretation and prediction of dynamical systems. It is a crucial component of earth sciences, particularly in numerical weather prediction (NWP). Data assimilation techniques have been widely investigated in NWP in the last two decades to refine the initial conditions of weather models by combining model forecasts and observational data. Most NWP centers around the world employ variational and ensemble-variational data assimilation methods, which iteratively reduce cost functions via gradient-based optimization. However, these methods require significant computational resources.
Recently, quantum computing has emerged as a new avenue of computational technology, offering a promising solution for overcoming the computational challenges of classical computers. Quantum computers can take advantage of quantum effects such as tunneling, superposition, and entanglement to significantly reduce computational demands. Quantum annealing machines, in particular, are powerful for solving optimization problems.
In a recent study, Professor Shunji Kotsuki from the Institute for Advanced Academic Research/Center for Environmental Remote Sensing/Research Institute of Disaster Medicine, Chiba University, along with his colleagues Fumitoshi Kawasaki from the Graduate School of Science and Engineering and Masanao Ohashi from the Center for Environmental Remote Sensing, developed a novel data assimilation technique designed for quantum annealing machines. “Our study introduces a novel quantum annealing approach to accelerate data assimilation, which is the main computational bottleneck for numerical weather predictions. With this algorithm, we successfully solved data assimilation on quantum annealers for the first time,” explains Prof. Kotsuki. Their study has been published in the journal Nonlinear Processes in Geophysics on June 07, 2024.
In the study, the researchers focused on the four-dimensional variational data assimilation (4DVAR) method, one of the most widely used data assimilation methods in NWP systems. However, since 4DVAR is designed for classical computers, it cannot be directly used on quantum hardware. Prof. Kotsuki clarifies, “Unlike the conventional 4DVAR, which requires a cost function and its gradient, quantum annealers require only the cost function. However, the cost function must be represented by binary variables (0 or 1). Therefore, we reformulated the 4DVAR cost function, a quadratic unconstrained optimization (QUO) problem, into a quadratic unconstrained binary optimization (QUBO) problem, which quantum annealers can solve.”
The researchers applied this QUBO approach to a series of 4DVAR experiments using a 40-variable Lorentz-96 model, which is a dynamical system commonly used to test data assimilation. They conducted the experiments using the D-Wave Advantage physical quantum annealer, or Phy-QA, and the Fixstars Amplify’s simulated quantum annealer, or Sim-QA. Moreover, they tested the conventionally utilized quasi-Newton-based iterative approaches, using the Broyden-Fletcher-Goldfarb-Shanno formula, in solving linear and nonlinear QUO problems and compared their performance to that of quantum annealers.
The results revealed that quantum annealers produced analysis with comparable accuracy to conventional quasi-Newton-based approaches but in a fraction of the time they took. The D-Wave’s Phy-QA required less than 0.05 seconds for computation, much faster than conventional approaches. However, it also exhibited slightly larger root mean square errors, which the researchers attributed to the inherent stochastic quantum effects. To address this, they found that reading out multiple solutions from the quantum annealer improved stability and accuracy. They also noted that the scaling factor for quantum data assimilation, which is important for regulating the analysis accuracy, was different for the D-Wave Phy-QA and the Sim-QA, owing to the stochastic quantum effects associated with the former annealer.
These findings signify the role of quantum computers in reducing the computational cost of data assimilation. “Our approach could revolutionize future NWP systems, enabling a deeper understanding and improved predictions with much less computational time. In addition, it has the potential to advance the practical applications of quantum annealers in solving complex optimization problems in earth science,” remarks Prof. Kotsuki.
Overall, the proposed innovative method holds great promise for inspiring future applications of quantum computers in advancing data assimilation, potentially leading to more accurate weather predictions.
About Professor Shunji Kotsuki
Dr. Shunji Kotsuki is currently a Professor at the Institute for Advanced Academic Research (IAAR), Chiba University, leading “Environmental Prediction Science.” He received his B.S. (2009), M.S. (2011), and Ph.D. (2013) degrees in civil engineering from Kyoto University. He has over 40 publications and received over 500 citations. Dr. Kotsuki is a leading scientist in data assimilation, deep learning numerical weather prediction with over ten years of research experience in the development of the global atmospheric data assimilation system (a.k.a. NICAM-LETKF). His research interests include data assimilation mathematics, model parameter estimation, observation diagnosis including impact estimates, satellite data analysis, hydrological modeling, and atmospheric and hydrological disaster predictions. He is currently the project manager for Goal 8 of Japan’s Moonshot Program, where he leads an interdisciplinary research team. This team includes experts in meteorology, disaster mathematics, information science, computer vision, ethics, and legal studies, all working together to achieve a weather-controlled society.
Funding:
This study was partly supported by the JST Moonshot R&D (JPMJMS2284, JPMJMS2389), JST PRESTO (JPMJPR1924), the Japan Society for the Promotion of Science (JSPS) KAKENHI grants JP21H04571, JP21H05002, JP22K18821, and the IAAR Research Support Program of Chiba University.
Reference:
Title of original paper: Quantum Data Assimilation: A New Approach to Solve Data Assimilation on Quantum Annealers
Authors: Shunji Kotsuki1,2,3, Fumitoshi Kawasaki4, and Masanao Ohashi2
Affiliations:
- Institute for Advanced Academic Research, Chiba University
- enter for Environmental Remote Sensing, Chiba University
- Research Institute of Disaster Medicine, Chiba University
- Graduate School of Science and Engineering, Chiba University
Journal: Nonlinear Processes in Geophysics
DOI: 10.5194/npg-31-237-2024
Contact: Shunji Kotsuki
Institute for Advanced Academic Research, Chiba University
Email: shunji.kotsuki@chiba-u.jp
Public Relations Office, Chiba University
Address: 1-33 Yayoi, Inage, Chiba 263-8522 JAPAN
Email: koho-press@chiba-u.jp
Tel: +81-43-290-2018
Recommend
-
An insect and machine enthusiast delves into the flight mechanism of living organisms: From the mosquito flapping to the high-performance drones
2024.03.12
-
Unfettered thinking, elaborate theorizing, and an accepting and positive attitude: With the precepts of his former supervisors in mind, Dr. Shimada is tackling the global food crisis
2023.01.04
-
Children-centered support and school operations: On the occasion of the founding of the Children and Families Agency
2023.02.15